Machine Learning, a Magical Black Box
As advertisers focus on the promise of efficiency gains from the implementation of machine learning algorithms, ignoring their limitations is problematic. More advertisers should be exploring a man plus machine framework - or considering whether machine learning is the right tool for their business at all. By understanding a little about how these models work, we can ensure we maximise their potential.
I went for a coffee with a former colleague and she asked me about my thoughts on a company that licensed machine learning software. Their offering and declared success rate sounded too good to be true and she was both intrigued and doubtful of the proposition. For me there is no doubt that machine learning provides a powerful weapon in an advertiser's arsenal but it is not necessarily the omnipotent offering often on sale.
Machine learning has different applications across industries, so for my colleague I will narrowly define its purpose in this digital context: machine learning enables the automation of programmatic media buying based on predicting an impression's ability to drive conversions or sales through the observation of existing data.
Machine learning techniques can be controversial among analysts. They abandon many of the traditional assumptions required for robust statistical modelling, instead utilising a technique of brute force. Typically a statistician would look at the desired conversion outcome, the type of variables they have to predict the outcome, and carefully select a model that best reflects these. For example if the desired outcome is binary or an amount, if the variables are linear/nonlinear or display collinearity, then the model choice would vary. Machines feel none of a statistician's woes about these model requirements. Rather than carefully selecting/deselecting the variables and identifying the correct model, machine learning runs the data through thousands of models and model iterations regardless of whether they meet the required assumptions or not. The single concern of this data crunching beast is which of these models yields the highest rate of accuracy when predicting the desired outcome.
From its definition and manner of execution we can examine a few things to scrutinise the next time the magical black box is offered to you:
Feeding the machine
As machine learning is using existing data, typically this will naturally have constraints on it. Perhaps to date you have only been targeting an audience of a certain profile. If you choose to scale your activity by opening up that audience or if over time your audience matures in understanding of your product, this universe will be altered and the machine learning approach you have developed may no longer apply. Essentially you will need to evaluate and 'maintain' the model continuously in order to ensure its optimal prediction power for an evolving audience.
Cause and effect
Machine learning uses observed data and may not indicate a causal relationship between your media activity and conversions. Any observations of performance e.g. a set of conditions or audience that yield a positive outcome, may suffer from self-selection bias and result in the active targeting of consumers who are already interested in your brand or product. There are different ways of tackling this but essentially determining the causality of the variables used to predict conversions is desirable and running over-arching control/exposed experiments to measure the impact of your creative and media can support this.
Accuracy and applicability
No matter how sophisticated your machine learning technique is, as with any modelling technique, accuracy is unfortunately not guaranteed. This could be for various reasons including that the inputs have low predictive value or sample constraints. What the "correct" level of predictive accuracy is will vary from brand to brand. Ultimately it needs to give you a better chance of success than your current optimisation technique and balance scale with a profitable ROI.
Once you are happy with accuracy, one of the big challenges of machine learning versus a traditional model is applicability. Its advantage over your traditional model is the increased chance of predictive accuracy but in abandoning all the normal assumptions required for model selection it is often no longer interpretable. In a traditionally developed model, should the model describe a mix of for example viewability, bid price and creative effectiveness, you can use it to say what combination of these variables will more likely result in a conversion. With machine learning the model will help you achieve accuracy in your predictions but the actual coefficients of the model are far less likely to offer you a meaningful description of the predictors e.g. it will be able to predict the likely value of an impression but not how to adjust your buy through viewability, bid-price and creative to yield the desired conversion outcome.
Man versus machine?
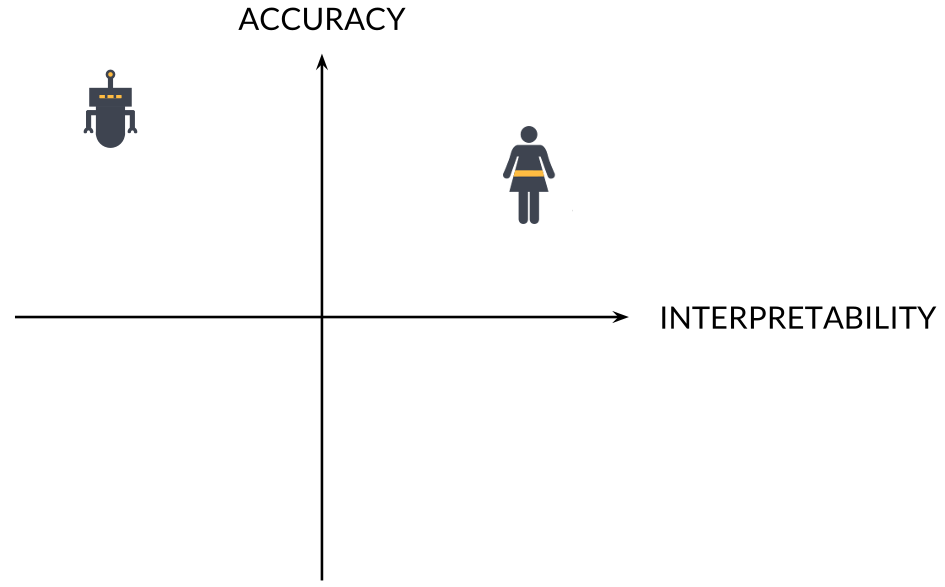
In summary machines possess the power to test models en masse with potentially a higher accuracy rate than even the most skilled statisticians. The model will be built within the confines of your historic creative strategy and data inputs. It will be complementary to, rather than a replacement of, causal experiments. Models produced through machine learning are indeed magical black boxes, and as such often devoid of interpretation. If you are able to apply the predicted value of an impression level directly to your programmatic buy, machine learning has the potential to offer a highly accurate assessment.
Regardless of your choice of model humans are still vital to this process. In contrast to machines they define the universe within which a model operates, identifying and potentially expanding the data set that feeds the machine. They can form hypotheses and design experiments to determine the causality of the media buys and the predictors of success. Or they can provide an alternative to machine learning altogether and create friendly, transparent models which allow you to interpret what is actually happening, offering valuable insight into what fuels success.
Personally, I would be cautious about swapping one out for the other.